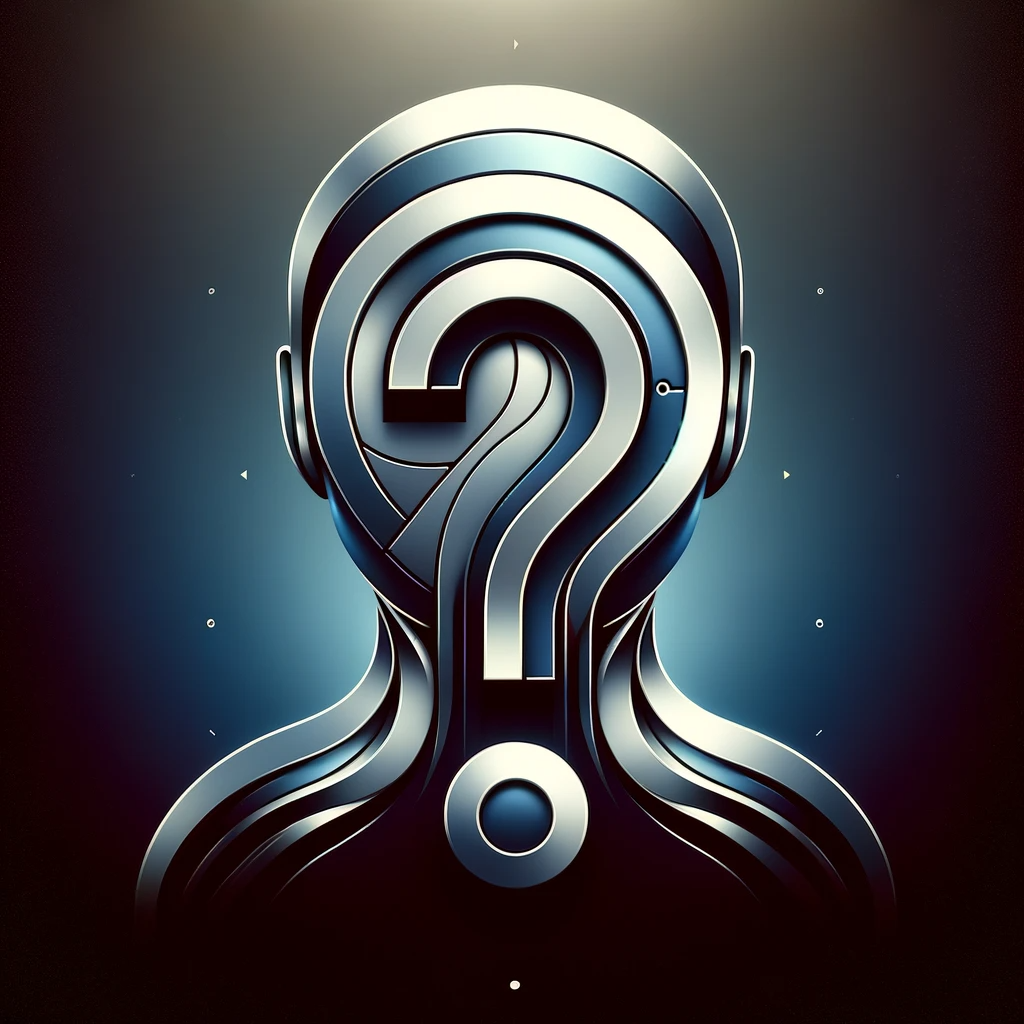
Each innovation wave is unique and distinct. Yet, many find it helpful to understand each wave through analogy, and I am no different. The most common analogies I’ve been considering with the current wave of generative AI are blockchain, self-driving cars, and Web 2.0. Here are some views on their similarities and differences, and how they each shape my thinking.
Blockchain
There are a lot of similarities between this era of generative AI and the Blockchain. Or, to be more precise, there are a lot of similarities between the way the tech world is reacting to these two different technologies.
At NextView, we were cautious about crypto and didn’t engage significantly. However, it was hard to ignore the volume of technical talent gravitating towards blockchain projects. Sometimes, the sheer influx of smart people into a trend can bring it into being. If nothing else, it definitely gave me pause and made my think that I might be missing something profound. Currently, it feels like every sharp technologist is pouring their effort into generative AI models or embedding them into their products—a movement too significant to overlook.
A less encouraging similarity is the deluge of capital and the suspension of disbelief in the AI space. Despite learnings from the latest tech bubble, investors are pouring immense funds into immature AI projects. Consequently, nearly every founder is attempting to weave an AI narrative into their ventures, regardless of its necessity or fit, which will likely lead to an unhealthy market environment and potentially drown out genuine opportunities.
The stark difference between crypto and AI lies in their use cases. Years into the crypto wave, many acknowledged the scarcity of practical applications. In contrast, generative AI has already inspired a myriad of compelling uses, and it’s easy to envision its integration across many categories of software. I’m very optimistic, but admittedly, gaps remain between what’s imaginable and what’s pragmatically useful today.
Which leads me to the second analogy.
Self Driving Cars
When Cruise automation was founded back in 2013, I remember that the thought of fully autonomous vehicles seemed like a science-fiction moonshot. Surely we were too far away for this to actually be a commercial reality anytime soon?
But then GM bought the company 3 years later, and suddenly, the zeitgeist changed. It seemed like the promise of self-driving cars was just around the corner. I remember telling my then 7-year old daughter that there was a great chance that she’d never need to learn how to drive because by the time she was of age, cars would just drive themselves. Incidentally, she will be starting drivers’ ed next year
Generative AI rhymes with self driving cars in that 1) it’s easy to envision a future where the technology is functional and 2) there are some serious gaps between the promise and reality. The first time you try to do anything with a generative AI product, it feels like magic. But you quickly also realize that in many areas, getting to a final usable outcome requires a lot more work than you expected. Also, the errors that you encounter (and the persistence of errors despite correction) makes it hard to fully trust the outputs without some layer of human oversight. To some degree, that’s the nature of a probabilistic model. But similar to a self-driving car, if you know the model does not always work, it’s hard to take your eye off the road and hands off the wheel.
What’s different from self-driving cars is that in a large proportion of use cases, the stakes aren’t nearly as high. Generative AI software is often not responsible for keeping a human alive in a metal box going at high speed in heavy traffic. Culturally, we are also in a place where self-driving cars need to perform well above the level of humans to be publicly accepted. In generative AI, this is not the case in many many areas. In some applications, the stakes of an error are relatively low as is the quality bar required to add value. In highly manual backoffice workflows, AI can significantly reduce the time required for many tasks while errors can be easily absorbed. In many of these cases, non-human level intelligence works just fine, because a human layer of oversight is maintained either for QA purposes or to take the work the final mile.
The other interesting similarity between self-driving cars and generative AI is that both lend themselves to speculating about second-order effects. When self-driving cars felt imminent, many pundits started thinking about its consequences on car ownership, housing choices, on-demand economies, etc. Similarly, conversations about generative AI very quickly move into speculation about the jobs that will be transformed or eliminated, the skills that future children will or won’t have to learn, and perhaps even the nature of work itself. There is something about the accessibility of the applications that spurs this kind of creativity, but also fear and concern for unintended consequences.
Web 2.0
The third analogy that I have used quite a bit when talking about generative AI is Web2.0. There are two reasons for this.
First, the ease at which developers can get started working with AI models is starting to lead to a Cambrian explosion of products and applications that leverage this technology. This is not dissimilar to the explosion of applications we saw in the mid-2000’s fueled by declining computing costs, cloud infrastructure, and social platforms.
Like Web2.0, many if not most of these startups are unlikely to get to significant scale. And both founders and investors are grappling with similar questions that were top of mind during early Web 2.0:
Are these features, products, or actual companies?
How defensible are these businesses? Is there any compounding advantage?
Is there a means of efficient distribution amidst the noise? Will new, native distribution channels emerge?
Can these businesses be built more efficiently than ever before, and what does that mean for how they will be financed?
These questions seem suspiciously familiar, and I wonder if the OG’s of the Web 2.0 wave like USV will have a distinct advantage at prosecuting this opportunity because of their embedded wisdom.
The other similarities with Web2.0 have to do with timing. Much like the early 2000’s, the market environment is fairly tepid given a prolonged period of weakness in startup financing activity and exits. As a result, even the most bullish investors are simultaneously licking their old wounds while trying to press forward aggressively. There is a familiar sense of both optimism for the long-term but fear in the short term given this context.
Web2.0 and Generative AI are also both application layer market surges created upon a prior period of infrastructure building. This may lead to interesting parallels. For example, in infrastructure building, the advantage tends to accrue to more experienced founders and teams that can garner the resources to build significant technology and sell to enterprises. It’s no surprise that in recent years, you heard multiple investors talk about how the profiles of founders they are backing are more experienced and more deeply technical than in the tail end of the last application wave. Is it possible that the pendulum will swing back towards possibly less seasoned but equally talented founders in the coming wave? I recall that during Web2.0, many VC’s had a hard time backing folks like Andrew Mason or Mark Zuckerberg or Drew Houston because of their relative lack of experience. Instead, you saw capital flow pretty smoothly to other founders that seemed more experienced on paper but had skills that were actually orthogonal to what was needed to build the Web2.0 space. Perhaps we’ll see the same thing as the most exciting builders in this new application renaissance look very different from the folks that had success building the infrastructure layer.
The other timing parallel between Web2.0 and generative AI is the earliness of both waves. While great companies were founded during Web2.0, one could argue that the best companies built on mobile, social, and the cloud emerged when the concept of Web2.0 was long gone. Indeed, I think most folks think of Web2.0 as a bit of a precursor to the golden age of software based businesses that emerged in the latter half of the 2010’s, a good 10 years after the Web2.0 application explosion began. Similarly, I think that it’s likely that the most interesting applications built on generative AI models are actually quite a few years out. Good companies will be built in the coming years, but they will likely pale in comparison to somewhat similar companies that will be built in 10 or 20 years.
There are other analogies that one could use to understand generative AI. Personally, I believe that this wave is much larger than the three I’ve mentioned above. Internally at NextView, we’ve talked about this wave of innovation wave in the same breath as the internet or the internal combustion engine, or even humanity’s ability to harness to electricity. It’s exciting to think about, but in some ways, these analogies are almost too grand to wrap one’s head around.
Finally, there is also the possibility that none of these analogies are apt because we are about to cross a species defining event horizon with AGI that will make the future impossible to fathom. That may very well be true, and if so, I hope that our AGI overlords don’t punish me for comparing them to Web 2.0